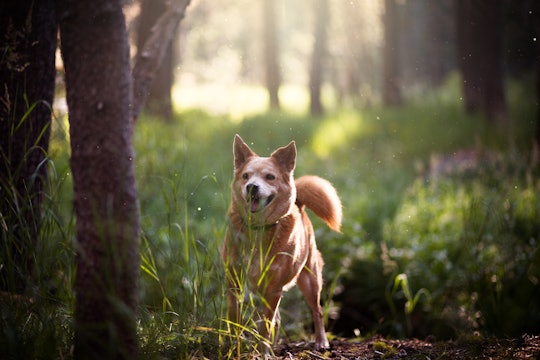
Photo by Cam Bowers on Unsplash
Dogs' medical records are helping machine learning researchers track ticks
Text classification turns tick talk into data that even a machine can understand
Spring came early this year, bringing warmth, flowers, longer days…and ticks. Ticks emerge from winter dormancy earlier in warmer years. Reported cases of tick-borne Lyme disease have increased over the past 25 years and expanded into new geographic regions as the planet warms. And humans aren’t the only ones who can catch Lyme. Our pets, especially dogs, are also at risk.
Getting hard numbers on ticks and where they are spreading isn’t straightforward. About 30,000 cases of Lyme in humans are reported to the Centers for Disease Control and Prevention (CDC) each year, which is only one tenth of what experts estimate the true number of diagnosed cases to be. To build models from incomplete data, public health officials pull from diverse sources: diagnostic laboratories, insurance claims, weather reports. Even infections in dogs can tell us something about infection rates in humans. These aren’t perfect methods, though, and they rely on people actively providing samples or undergoing somewhat invasive tests.
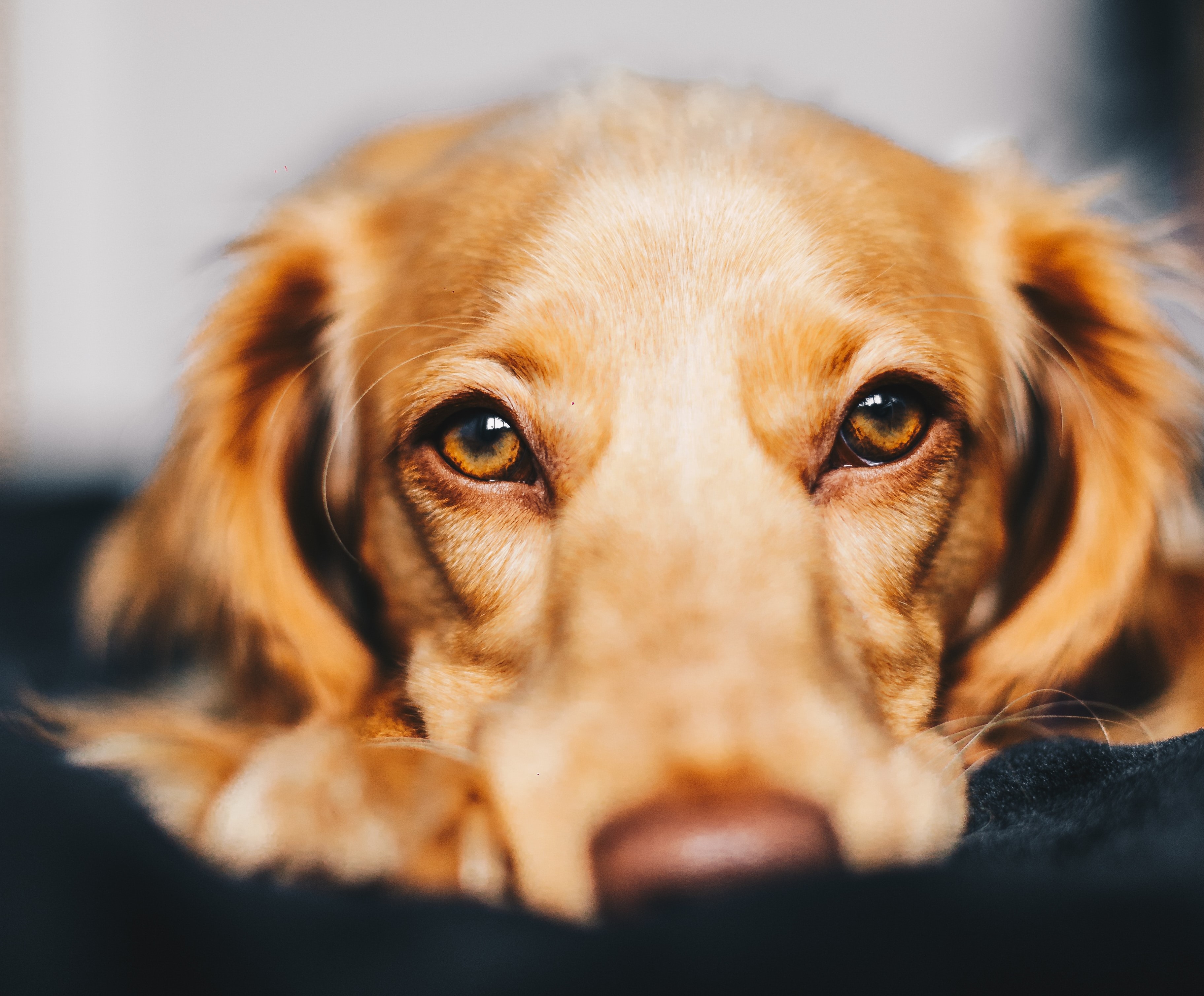
Your dog's medical records could help science
Photo by Ryan Walton on Unsplash
Luckily, there might be another option for detecting ticks. A cross-disciplinary team led by James O'Neill at the University of Liverpool recently presented a method to use machine learning to predict ticks’ presence from a pet's health records.
In order to build the method, O’Neill and his coauthors took advantage of the Small Animal Veterinary Surveillance Network, an initiative that has accumulated over 3 million records from veterinary visits in the UK. These records include “clinical narratives” — written descriptions of veterinarians' observations. These notes are rich with information about pet health. But it isn’t simple for a computer to make sense of them. Human mistakes like misspellings and transcription errors can make normal words incomprehensible to a machine. Medical jargon is also often missing from the dictionaries that language processing tools rely on.
To get around these problems, the authors built up levels of meanings for sub-words, words, and combinations of words within the text. They had to create a sort-of dictionary for the algorithm to refer back to when looking for evidence of ticks. Assigning classifications at different levels allowed the machine learning algorithm to recognize patterns, even when the words or phrases didn’t exactly match the examples in the dictionary. For example, "outdoors" and "outside" might be synonyms to a reader but not to a computer. A computer can be trained to recognize the sub-word "out." If being outside increases a dog's chance of getting a tick, the computer can assign higher tick probability to "out" when it is in the same sentence as, say, "walk," and a lower tick probability when it shows up as part of a common and unrelated word like "about."
Cn y rd ths? Most likely you can. There's a lot of information in text that isn't strictly necessary for our minds to understand it. We subconsciously recognize the important patterns and ignore the rest. In this machine learning algorithm, the computer did something like the reverse of what your mind just did. It took the vet records as input, simplified the text by identifying the important features (possible key words like "outside," for example), did this multiple times creating different layers, and then spat out a probability that a tick was present on an animal.
The particular method used here is called a "convolutional neural network," a type of machine learning that got its name because it handles data similarly to how neurons in the eye pick out patterns in visual information. The Liverpool group tested variations on the method, allowing the program to more easily forget the features it identified, and to more easily remember information from layers farther back in the process. Their best version was 84% accurate overall, but only 72% accurate when specifically predicting an absence of ticks.
If 72% accuracy seems low, that's because machine learning isn’t yet ready to diagnose your pet. Artificial intelligence is just starting to be used to diagnose medical images, aid drug discovery, and prevent surgical complications. The method of tick detection described here was only presented at a conference, so the findings should be taken with a grain of salt for now. There’s still a high rate of false negatives with the method the researchers described. Partly this is because there are far more vet records without ticks than with, making comparisons unbalanced. Another shortcoming is that the method only reports tick presence, whereas a blood test can provide information about tick-borne disease directly. Used carefully, however, text mining of dogs’ medical records can be a complementary tool for understanding tick ecology.
.png)
An Asian longhorned tick, a species that was first reported in the US in 2017.
Ticks are changing their behavior and their range in response to changing climates. We can forecast where ticks and tick-borne diseases will be based on where they are now and the environments they prefer, but our predictions are only as good as the data they’re based on. Veterinary clinical narratives offer a complementary source of information to add to our current data. In fact, positive blood tests in vet records between 2001 and 2007 clued scientists in to the possible existence of a not-yet discovered pathogen in Wisconsin and Minnesota. Scientists knew that a bacterium called Ehrlichia chaffeensis could infect dogs, but the particular species is rare in that region of the US. Yet, dogs tested positive at a surprisingly high rate for antibodies associated with the disease, which could happen if the dogs had been exposed to a similar species. Sure enough, human cases of ehrlichiosis were discovered in Wisconsin and Minnesota in 2009, and a new species, Ehrlichia muris euclairensis, was formally described in 2011.
This is a really nice breakdown of how we can use machine learning to help us transform otherwise unwieldy data sources into something usable. In particular, there is such valuable information in long form clinical records, but variation in communication styles, abbreviations, etc. are rampant. This is also a great example of the One Health framework: understanding the health of other animals can help us better understand our own.